The industrialisation of data science is something that interests many companies these days. But it is not simply a technical topic: IT, business and organisational set-up must be addressed at the same time. In this chapter, we will introduce you to ML Ops, and the challenges of this approach.
A few decades ago, data science was a purely theoretical discipline, studied mainly in academia and research teams. Today, the business world invests in data science projects with the aim of creating value.
This requires a significant maturity in operational processes, which were built with traditional IT system requirements in mind, but unfortunately less suited for machine learning models.
50%
A study conducted by Algorithmia shows that half of the companies spend between 8 and 90 days to implement a single AI model into production. This considerable time is due to constraints which slow down the production process.
The ML Ops approach allows businesses to respond to these challenges in a more timely manner.
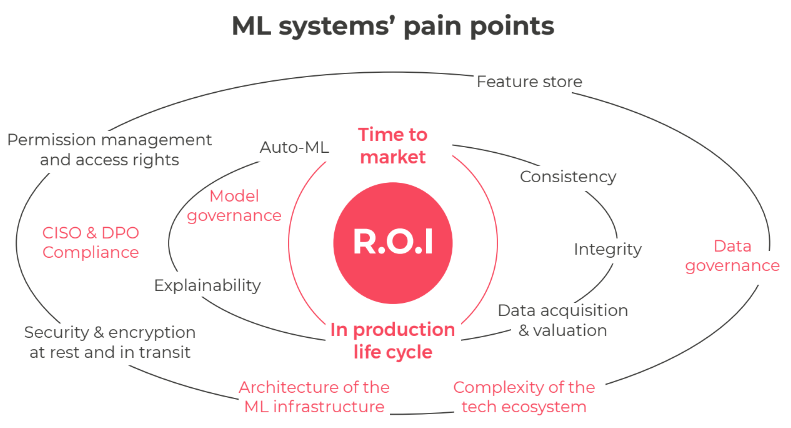
- What is ML Ops?
- ML Ops at the intersection of several areas
- Challenges of a ML approach
All those topics are answered in the E-Book
To learn more about it Download it here